
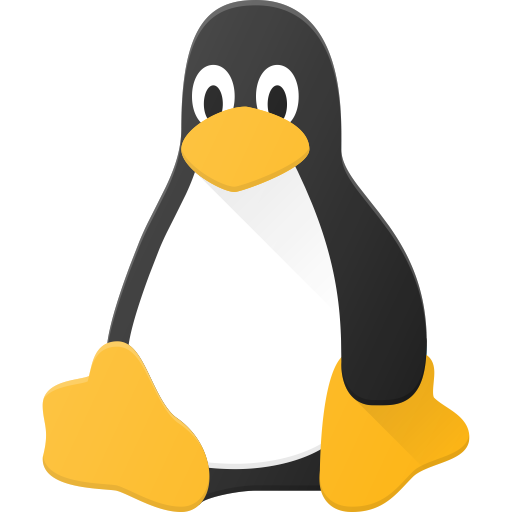
That was the my first distro. Getting it to run off a FireWire drive was an interesting introduction to Linux.
Fun fact: yum stands for Yellow dog Update Manager. I know it’s been replaced by dnf but I still think that’s cool.
That was the my first distro. Getting it to run off a FireWire drive was an interesting introduction to Linux.
Fun fact: yum stands for Yellow dog Update Manager. I know it’s been replaced by dnf but I still think that’s cool.
And you can’t tell when something is active/focused or not because every goddamn app and web site wants to use its own “design language”. Wish I had a dollar for every time I saw two options, one light-gray and one dark-gray, with no way to know whether dark or light was supposed to mean “active”.
I miss old-school Mac OS when consistency was king. But even Mac OS abandoned consistency about 25 years ago. I’d say the introduction of “brushed metal” was the beginning of the end, and IIRC that was late 90s. I am old and grumpy.
Say all you want about hallucinations, but AI will never be able to outperform humans at bullshitting, so sales and marketing is safe.
“It’s popular so it must be good/true” is not a compelling argument. I certainly wouldn’t take it on faith just because it has remained largely unquestioned by marketers.
The closest research I’m familiar with showed the opposite, but it was specifically related to the real estate market so I wouldn’t assume it applies broadly to, say, groceries or consumer goods. I couldn’t find anything supporting this idea from a quick search of papers. Again, if there’s supporting research on this (particularly recent research), I would really like to see it.
If there is any research from the last 50 years suggesting this actually works, I’d love to see it.
I haven’t seen this movie in like 25 years, but I still read this in Marisa Tomei’s voice.
Wait, isn’t it the other way around? You should arrive in NY earlier than you left London, since NY is 5 hours behind London. So if you leave at 8:30 and arrive 1.5 hours later, it should only be 5AM when you arrive.
You might need a third breakfast before your elevenses in that case.
Jerboa is solid, but it’s not feature-rich. Not great for media browsing. It’s still my main client since I use Lemmy mostly for text, not images or videos.
Eternity and Voyager are worth looking at, too.
Interesting read, thanks! I’ll finish it later, but already this bit is quite interesting:
Without access to gender, the ML algorithm over-predicts women to default compared to their true default rate, while the rate for men is accurate. Adding gender to the ML algorithm corrects for this and the gap in prediction accuracy for men and women who default diminishes.
We find that the MTEs are biased, signif-icantly favoring White-associated names in 85.1% of casesand female-associated names in only 11.1% of case
If you’re planning to use LLMs for anything along these lines, you should filter out irrelevant details like names before any evaluation step. Honestly, humans should do the same, but it’s impractical. This is, ironically, something LLMs are very well suited for.
Of course, that doesn’t mean off-the-shelf tools are actually doing that, and there are other potential issues as well, such as biases around cities, schools, or any non-personal info on a resume that might correlate with race/gender/etc.
I think there’s great potential for LLMs to reduce bias compared to humans, but half-assed implementations are currently the norm, so be careful.
Being factually incorrect about literally everything you said changes nothing? Okay.
More importantly, humans are capable of abstract thought. Your whole argument is specious. If you find yourself lacking the context to understand these numbers, you can easily seek context. A good starting place would be the actual paper, which is linked in OP’s article. For the lazy: https://www.nature.com/articles/s41598-020-61146-4
It’s 14,000 to 75,000, not millions.
Microplastics are in the range of one micrometer to five millimeters, not nanometers.
They link to the full source paper: https://www.nature.com/articles/s41598-020-61146-4
That seems more like your problem than OP’s.
After all these years, I’m still a little confused about what Forbes is. It used to be a legitimate, even respected magazine. Now it’s a blog site full of self-important randos who escaped from their cages on LinkedIn.
There’s some sort of approval process, but it seems like its primary purpose is to inflate egos.
It was an SEO hellhole from the start, so this isn’t surprising.
Do Forbes next!
I think there are two problems that make this hard to answer:
Not all sentences that can be parsed grammatically can also be parsed logically.
Human-language sentences do not contain all the information needed to evaluate them.
It is impossible to fully separate context from human language in general. The sentence “it is cold” is perfectly valid, and logically coherent, but in order to evaluate it you’d need to draw external information from the context. What is “it”? Maybe we can assume “it” refers to the weather, as that is common usage, but that information does not come from the sentence itself. And since the context here is on the Internet, where there is no understanding of location, we can’t really evaluate it that way.
It’s hot somewhere, and it’s cold somewhere. Does that mean the statement “it is cold” is both true and false, or does that mean there is insufficient information to evaluate it in the first place? I think this is largely a matter of convention. I have no doubt that you could construct a coherent system that would classify such statements as being in a superposition of truth and falsehood. Whether that would be useful is another matter. You might also need a probabilistic model instead of a simple three-state evaluation of true/false/both. I mean, if we’re talking about human language, we’re talking about things that are at least a little subjective.
So I don’t think the question can be evaluated properly without defining a more restrictive category of “sentences”. It seems to me like the question uses “sentence” to mean “logical statements”, but without a clearer definition I don’t know how to approach that. Sentences are not the same as logical statements. If they were, we wouldn’t need programming languages :)
Apologies for the half-baked ideas. I think it would take a lifetime to fully bake this.
It says:
Available Architectures
aarch64, x86_64
And it uses Android Translation Layer. Interesting. I’ll give it a shot on my desktop later.
I feel this. I use Waistline. Or I should say I would use Waistline if it wasn’t such a drag, but in reality I haven’t launched it in months. It was the closest drop-in replacement I could find for MyFitnessPal (which is proprietary and extraordinarily bloated), letting me search a database of foods either by type or barcode. But MyFitnessPal was a much smoother experience. I still recommend Waistline because AFAIK it’s the best out there, but the bar is pretty low.
Both have a problem with redundant and contradictory items in the database, because they are at least partly crowdsourced. Lots of entries have weird or meaningless units.